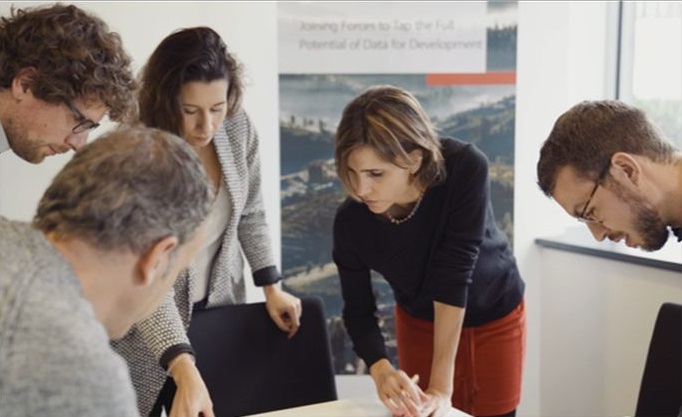
22.07.2020
‘Looking for what makes the difference’
The GIZ Data Lab analyses statistical outliers in data sets to find innovative solutions – a model that promises to provide insight into the COVID-19 pandemic too.
Can you measure the impact of a project using mobile communications data? Or use satellite images to record data? And can you harness artificial intelligence to advise smallholder farmers? The Deutsche Gesellschaft für Internationale Zusammenarbeit (GIZ) GmbH is examining these and other similar questions in its Data Lab, which was set up in 2019. The lab is now using the data-powered positive deviance approach to test a procedure that turns current methods on their head.
Data Lab coordinator Catherine Vogel and advisor Andreas Glücker explain how, instead of excluding statistical outliers in data sets as researchers normally do, they specifically look for them. This is based on the conviction that these ‘positive deviants’ can provide considerable added value for development cooperation – and also in the context of the current COVID-19 crisis.
What is the thinking behind the positive deviance approach and why is GIZ looking at it?
Catherine Vogel: Data sets are usually used to determine average values for the behaviour of groups or individuals. Any statistical outliers are often ignored. Yet unusual, positive deviants in the data show that there are individuals or groups that achieve better results than others, even though the conditions and challenges are similar. We might be looking at villages whose rice harvest is higher than in other places with similar farming conditions, city districts with comparatively little crime, or hospitals that still manage to provide adequate care even during pandemics. Our pilot projects are designed to help us understand what types of behaviour make these unusual achievements possible and whether they can be transferred. The aim is to find unusual and innovative solutions to foster this kind of deviance. The positive deviance approach was established back in the 1990s; our project is now harnessing the opportunities provided by big data to identify positive deviants.
What are the challenges here?
Catherine Vogel: The biggest obstacle in analysing positive deviance is the availability of data. We need current, comprehensive data in order to draw reliable conclusions about positive deviants. Digital sources such as satellite images and mobile communications data offer great potential but are not always accessible. This is where we cooperate with international partners. The next big task involves confirming that outliers found in the data actually exist in practice and then looking for what makes the difference. If we manage to do that, we will have made great progress in harnessing local creativity much more effectively.
How has the technique been put into practice?
Andreas Glücker: We are currently using the positive deviance approach in seven different countries and cooperating closely with the projects there. In Ecuador, we are looking for farmers who have been working within the Amazon region for many years and, in contrast to widespread practice, do not clear the land. In Somaliland, we are trying to find positive types of behaviour that enable cattle herders to cope with periods of drought without suffering major losses to their herds. In all these projects, considerable added value is created by combining digital, quantitative data with extensive expertise on local conditions, which comes mostly from the GIZ projects involved.
How could this method be used to help understand the spread of coronavirus better?
Catherine Vogel: During the coronavirus crisis, we have been looking for the districts in Germany that have managed to reduce the rate at which COVID-19 is spreading much faster than we would normally expect. And we have in fact managed to identify districts that have fared better than others even after all the known positive factors have been taken into account. These developments are extremely interesting and provide new insight into particular types of behaviour during a pandemic.
How do you go about doing that? Are any results available yet?
Andreas Glücker: The first step is to ensure that results are comparable to make sure that we are not comparing the proverbial apples and oranges. So we grouped districts according to factors such as population density. Then we used mobility patterns based on mobile communications and weather data to train an artificial intelligence model. Once the model was ready, we used it to predict the spread of the virus in each case. If the actual values reported by the Robert Koch Institute for a particular district are considerably better than the value we predicted, that suggests that there are factors or patterns of behaviour that have not yet been identified. These then obviously have to be examined in more detail. We used this approach in a sustainability hackathon organised by Siemens and won the Sustainability Impact prize.
And where do you go from here?
Catherine Vogel: The results of the COVID-19 pilots are promising, and we will be taking a closer look at the districts that have been identified. And our other approaches also have great potential for development cooperation in that they provide a method that begins by looking for existing solutions before trying to reinvent the wheel.